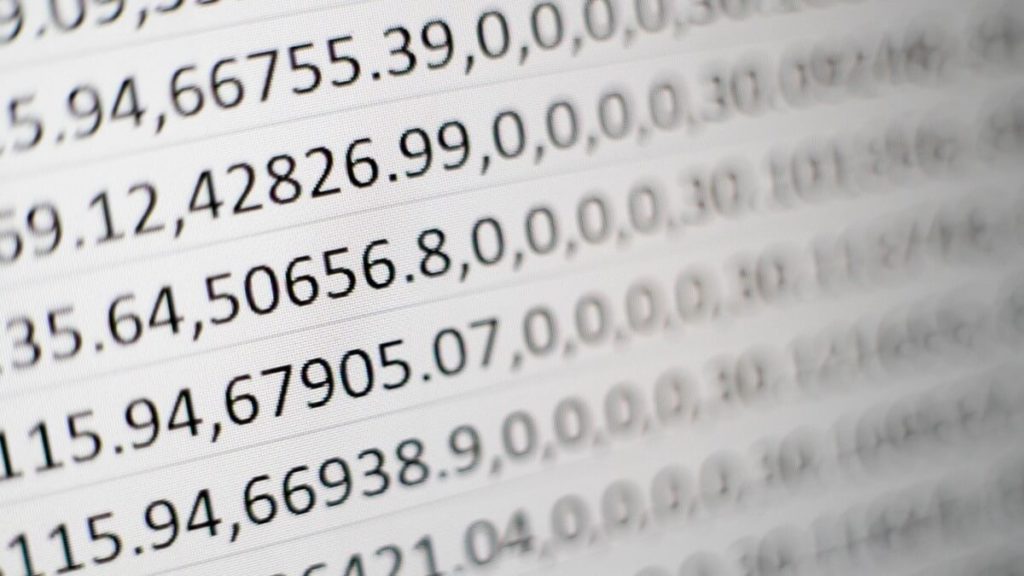
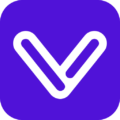
HYPERVSN
Marketing Department
Exploring the Diverse Forms of Big Data Analysis
The diverse forms of big data analysis are crucial for marketing because they provide a comprehensive toolkit for understanding customer behaviour, optimising strategies, predicting trends and prescribing actionable insights. By leveraging these various forms of analysis, marketers can make informed decisions, tailor their approaches and stay agile in a rapidly evolving marketplace, ultimately enhancing the effectiveness of their marketing efforts.
Big data analysis has become an indispensable tool in today’s data-driven world. As businesses and organisations grapple with vast and complex datasets, various forms of big data analysis have emerged to extract valuable insights and drive informed decision-making. Let’s explore several forms of big data analysis, shedding light on their unique characteristics and applications.
Descriptive analytics
Descriptive analytics is the foundation of big data analysis. It involves the examination of historical data to understand what has happened in the past. This form of analysis provides valuable context and helps businesses identify trends and patterns. Descriptive analytics is often used in business intelligence dashboards to create visual representations of data, making it easier to comprehend complex information. For instance, an e-commerce company might use descriptive analytics to track sales trends over the past year, visualising monthly revenue and identifying peak shopping periods.
Diagnostic analytics
Diagnostic analytics goes beyond describing what has happened and aims to uncover why it happened. It involves a deeper investigation into data to identify the root causes of specific events or trends. Businesses can use diagnostic analytics to understand why sales declined during a particular quarter or why customer churn rates increased. By pinpointing the underlying causes, organisations can make targeted adjustments to their strategies or processes to address these issues effectively.
Predictive analytics
Predictive analytics leverages historical data to forecast future outcomes or trends. This form of analysis uses statistical techniques, machine learning algorithms and data modelling to make predictions. For example, a weather forecasting service uses predictive analytics to generate forecasts based on historical weather data and current atmospheric conditions. In the business world, predictive analytics can be used to forecast customer demand, identify potential risks, and optimise supply chain operations.
Prescriptive analytics
Prescriptive analytics takes big data analysis a step further by not only predicting future outcomes but also recommending specific actions to achieve desired results. It provides actionable insights that guide decision-making. For instance, in healthcare, prescriptive analytics can help doctors determine the most effective treatment plan for a patient based on their medical history and current condition. In supply chain management, it can recommend optimal inventory levels and shipping routes to minimise costs while meeting customer demand.
Text analytics
Text analytics, also known as text mining or natural language processing (NLP), focuses on extracting valuable insights from unstructured text data. This form of analysis is particularly valuable for businesses dealing with customer reviews, social media conversations, emails, and other text-based sources. Text analytics can identify sentiment, key topics, and emerging trends within large volumes of text data. For example, a restaurant chain can use text analytics to analyse customer reviews and identify recurring themes or complaints to improve customer satisfaction.
Social media analytics
Social media analytics specifically focuses on data generated through social media platforms. It involves monitoring and analysing social media conversations, user engagement and trends. Companies use social media analytics to gauge brand sentiment, measure the impact of marketing campaigns and identify influential social media users. For instance, a cosmetics brand can analyse social media engagement to determine which products are gaining popularity among influencers and consumers, informing their marketing and product development strategies.
Geospatial analytics
Geospatial analytics combines geographic data with big data analysis to gain insights into spatial patterns and relationships. It is particularly valuable for businesses in transportation, urban planning and logistics. For example, a ride-sharing company uses geospatial analytics to optimise driver routes, allocate resources efficiently, and predict demand in different areas based on historical location data.
Conclusion
The forms of big data analysis mentioned above represent a diverse spectrum of techniques and approaches that cater to different business needs and objectives. Whether it’s gaining insights from historical data, diagnosing the reasons behind certain trends, predicting future outcomes, prescribing optimal actions, extracting valuable information from unstructured text, monitoring social media interactions, or leveraging geographic data, big data analysis is a versatile and indispensable tool that empowers businesses and organisations to make data-driven decisions and unlock the full potential of their data resources. As technology and data continue to evolve, so too will the forms and capabilities of big data analysis, shaping the future of data-driven decision-making across various industries.
Related Articles
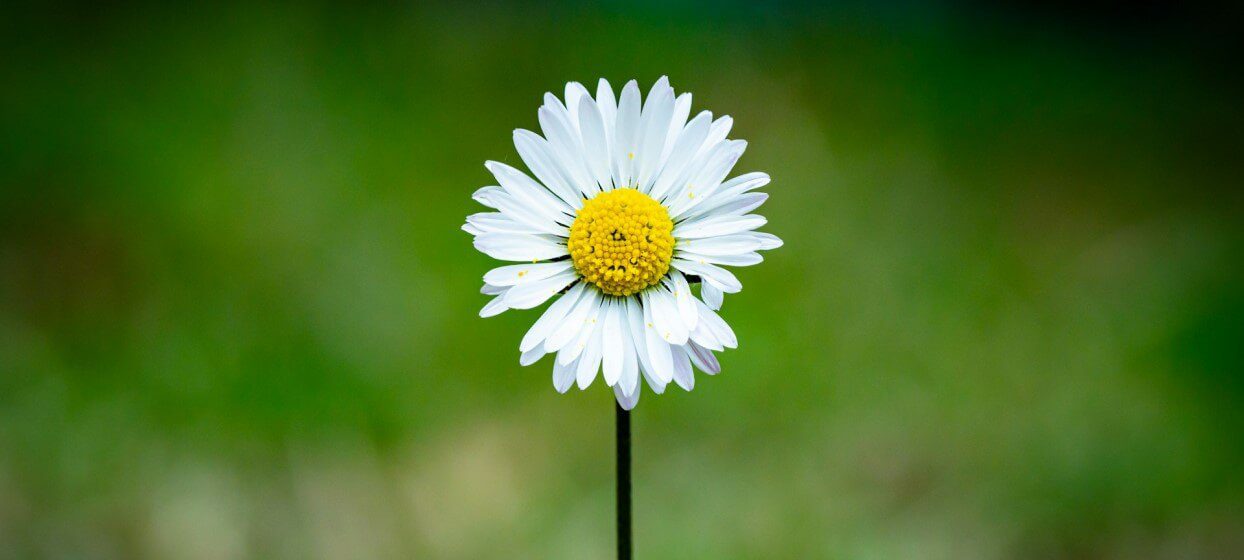
The Rise of Sustainable and Ethical Consumption
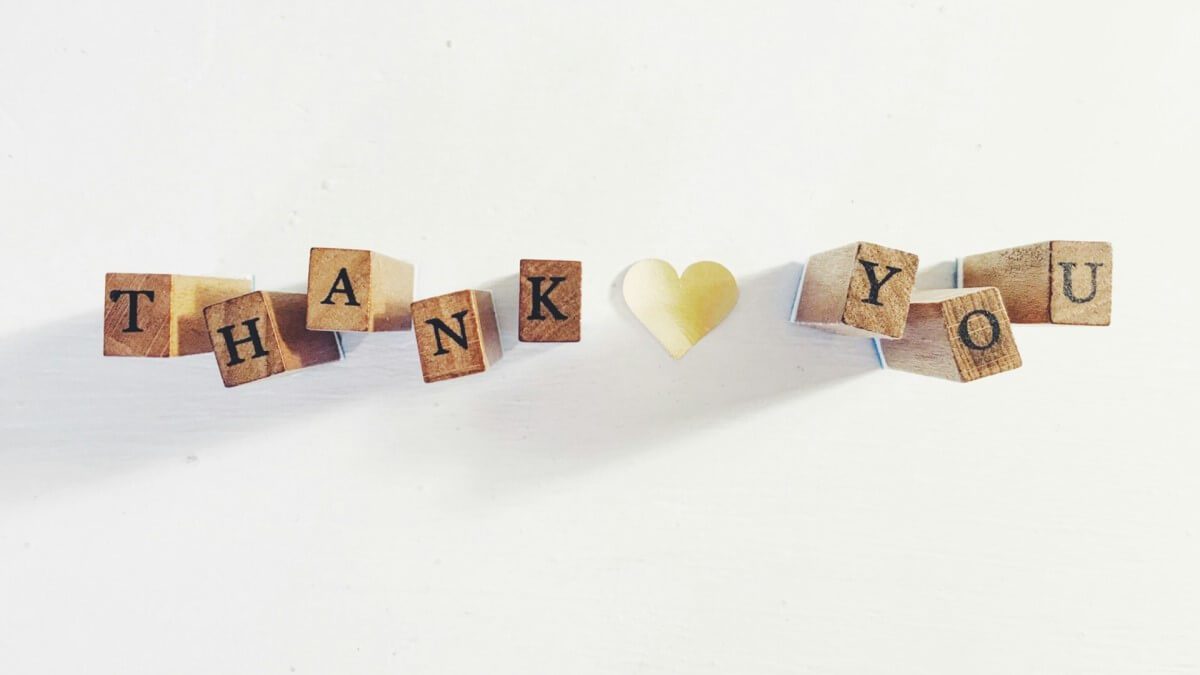
Elevating Thank You Pages to Creative Heights
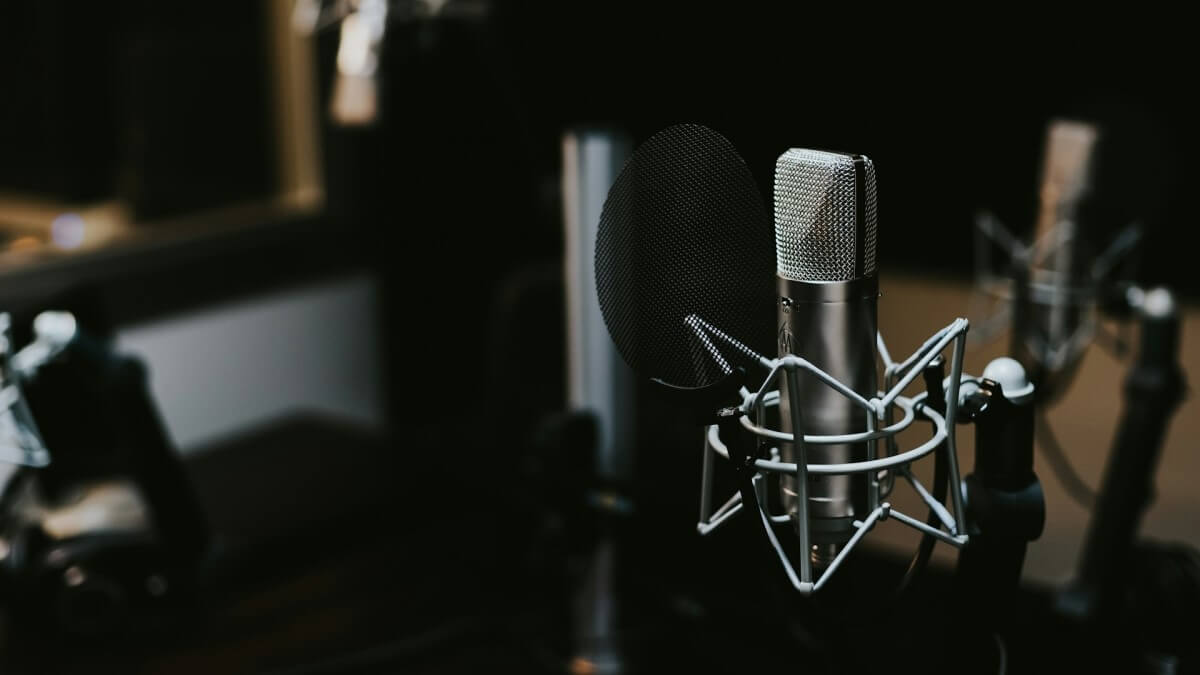
Product Podcasts and Their Significance with Younger Audiences
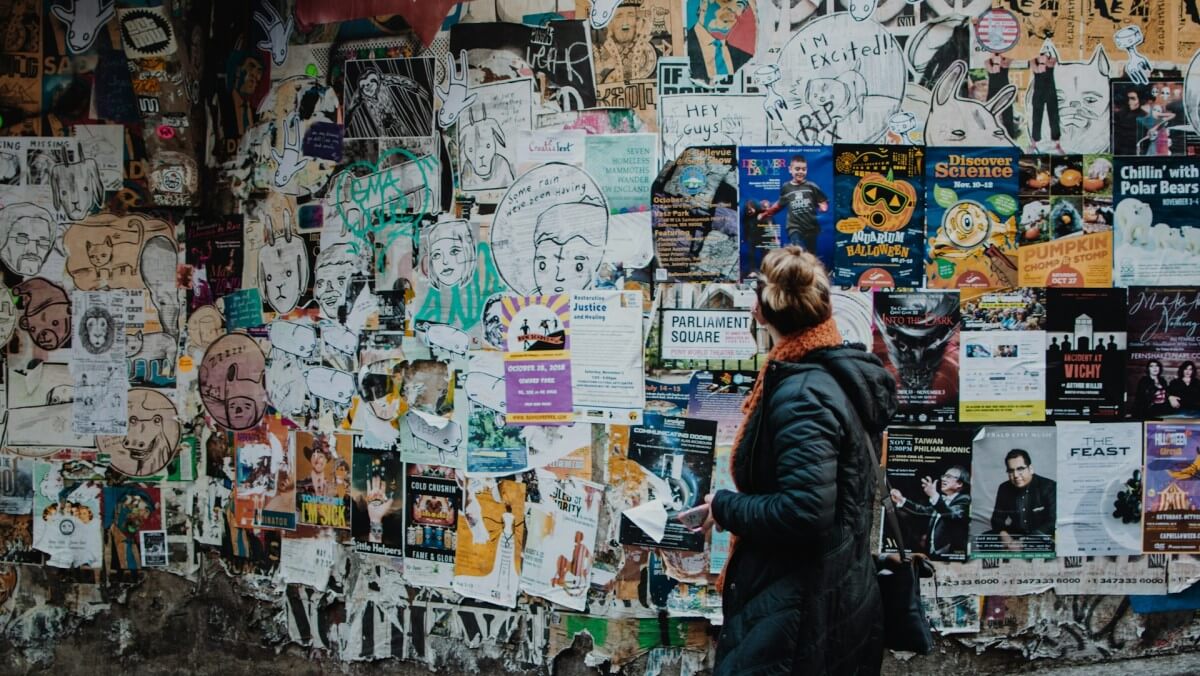
Innovative Strategies for Diversifying Advertising
subscribe
Join our mailing list to keep up with the latest HYPERVSN news
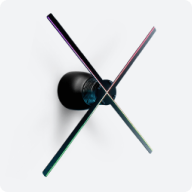
HYPERVSN 3D Catalog
USD 1,200
Qty
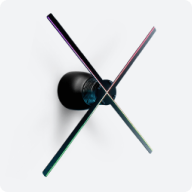
HYPERVSN 3D Catalog
USD 1,200
Qty
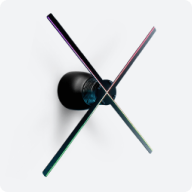
HYPERVSN 3D Catalog
USD 1,200
Qty
Cart Subtotal:
USD 3,600Request a Callback
☎️